
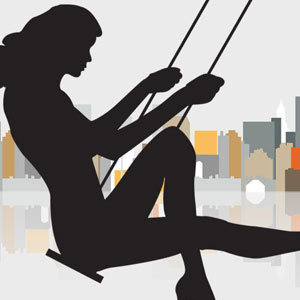
Cohen - CEREMADE, UMR CNRS 7534, Université Paris DauphineĪbstract: Tubular and tree structures appear very commonly in biomedical images like vessels, microtubules or neuron cells.
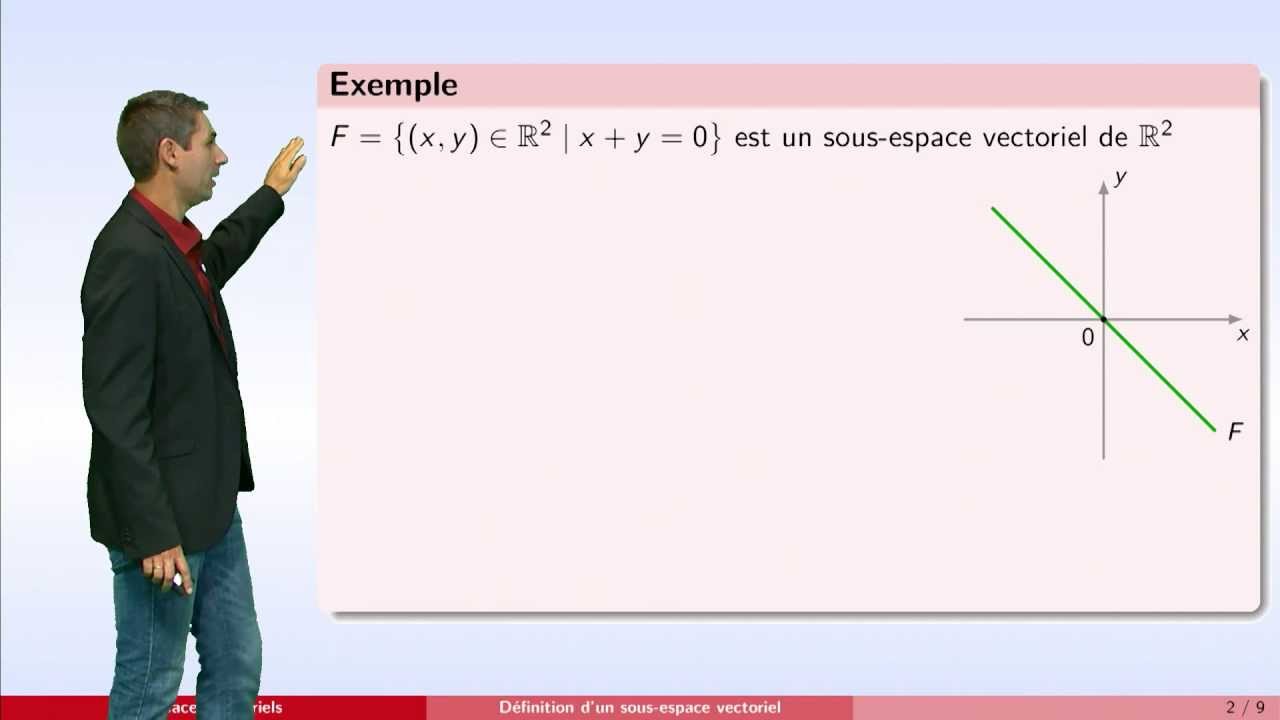
Geodesic methods for Biomedical Image Segmentation I'll briefly demonstrate a way to detect repetitive patterns using this framework. Such energies facilitate a kind of "hierarchical MDL" criterion and should be useful in detecting multiple objects, motions, homographies, and much more.
#Hyperplan vectoriel how to#
I'll talk about how to do better using a "2-level MRF" defined over "super-labels." The second part of the talk will present some unpublished work on energies with a hierarchy of "label costs" and how to optimize them effectively. assumption is inappropriate for generating an image, then it is equally inappropriate for generating complex objects *within* the image (person, car), and yet this is what the standard setup entails. and this is simply not a good model for natural images. Everyone agrees that the MRF aspect is important, because without it the pixels are assumed i.i.d. Super-Labels and Hierarchical Label CostsĪndrew Delong - University of Western OntarioĪbstract: The first part of the talk will be about a simple segmentation functional similar to Boykov-Jolly / GrabCut, The standard setup includes a GMM for foreground label, another for the background, and an MRF for regularization. Taken together, these three pieces constitute the first system for truly "end-to-end" learning of image segmentation, where all parameters in the algorithm are adjusted to directly minimize segmentation error. I will also present new work in each of these areas: 1) a segmentation algorithm based on convolutional networks as boundary detectors, 2) the Rand index as a measure of segmentation quality, and 3) the MALIS algorithm, based on ultrametric learning, for training boundary detectors to optimize the Rand index segmentation measure. Such algorithms have three basic components: 1) a parametrized function for producing segmentations from images, 2) an objective function that quantifies the performance of a segmentation algorithm relative to ground truth, and 3) a means of searching the parameter space of the segmentation algorithms for an optimum of the objective function. Supervised machine learning is a powerful tool for creating accurate image segmentation algorithms that are well adapted to any dataset. In this talk, I will present a new machine learning method for the supervised learning of image segmentation. And high-throughput, highly-accurate, automatic image segmentation is the most important technology for the success of connectomics. It is still early days and much effort is still dedicated to developing good methods for mapping neural networks. Srini Turaga - Sebastian Seung's lab at MITĪbstract: Connectomics is a new research effort in neuroscience dedicated to mapping the connectivity of real biological neural networks in the brain. "End-to-end" machine learning of image segmentation (for Connectomics) LIGM - Equipe A3SI : seminaire de recherche Séminaire de recherche A3SI
